When we test new tools, whether in marketing or in our own lives, it’s always important to follow the scientific method and test distinct cohorts against one another. Having a clear understanding of the difference between your control and test groups within your experiment is the best way to learn from your results.
This can lead people managing campaigns for themselves or agencies working to drive profitable performance for clients, to feel stuck at a crossroads. Do we embrace this new change, or wait until we understand it a little better, at the risk of missing that exciting “early adopter” stage of innovation implementation?
Then, when we design new tests before launching new tools, it’s common to do so based on the recommendations of their progenitors, the creators of the tools. Why wouldn’t we just follow the instructions? Well, at the core of all this new marketing technology is one singular motive. Whether it’s Google, Microsoft, or any of the other major players creating new tools to optimize our campaigns, they all seek to optimize their own campaigns and generate profit. Profit for themselves, built on the backs of marketing experts and agencies with goals of their own.
Broad match isn’t the first new tool that Google has introduced, and it won’t be the last. Even within the scope of Google keywords, we can see the pattern of iterations based on the same objective. To show ads to more people. It goes without saying that this will align in many ways with our objectives and our clients’. But ultimately tools like these have the potential to run rampant. When this happens, they run the risk of wasting money due to ineffective campaigns built to the letter based on the instructions and recommendations of the creators of the tool in the first place. Instructions built to further their objectives before our own.
In the case of broad match, these keywords have the potential to expand our campaigns while leveraging cutting-edge technology to capture search terms in an efficient and effective way. But they don’t always do this. Even when they do, they’ve been proven to reduce the ability of other keywords such as phrase and exact to continue building on proven successful efforts.
Google’s recommendation is generally to just add broad match keywords to your existing campaign structure. This creates campaigns where broad, phrase and exact all coexist within ad groups. However, when tested, we saw a clear shift in proportional spend at the keyword level that indicated Google’s algorithm was favoring traffic sent to broad match keywords over their phrase and exact counterparts.
Over the course of three months, broad match went from 11% of total spending to 52% of total spend, a 372% relative increase in spend! At the same time, both phrase and exact match keyword spend decreased. This was when we decided it was time to stop this pattern from accelerating further, and designed our own optimized structure for broad match testing.
After waiting for the data to mature, the results of this new structure were overwhelmingly positive. This blog post will cover the impact of our best practice broad match testing structure and highlight its efficiency in reducing broad match overlap and improving aggregate performance. We also introduce a reconfigured Growth Efficiency Index (GEI) metric in order to gauge campaign scaling efficiency and measure our results. These are the tools that will allow you to test the efficacy of broad match keywords within your Google ads campaigns. As an added bonus, the concepts we used in designing our testing process can even be applied to any other fancy new toys yet to come for the world of digital marketing.
How Did We Get Here?
Check out our previous post which spurred this test. It’s not required reading in order to understand the concepts covered here, but it goes into further detail on how the initial structure of our campaigns was determined, and how you can set that up yourself. To briefly summarize, we saw that broad match wasn’t doing what Google told us it would, and designed a new campaign structure in order to help it reach its true potential.
We created the campaign structure below, and applied it to multiple non-brand search campaigns for a national retail client, spending roughly $50K over the course of six months.
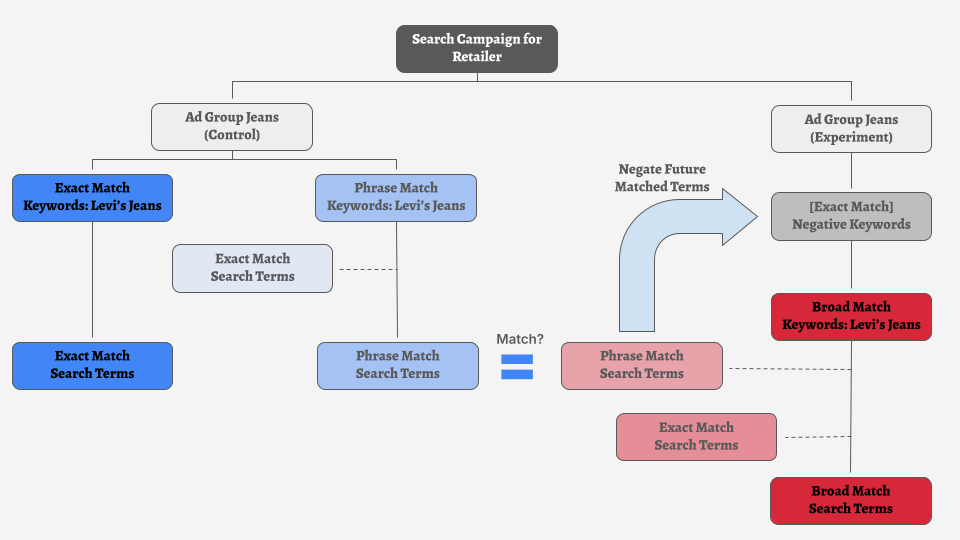
One of the risks inherent in this structure is that we were going to be seeing performance come in from these broad match keywords, and then immediately adding in negatives that prevent the next day’s campaign spend from doing what it did yesterday.
So here’s our hypothesis: Automated negation of search terms delivered by broad match keywords that can be delivered by phrase and exact keywords instead will not stop all delivery of our broad match cohort. If anything, we thought we would see an improvement in the way broad match would perform thanks to these “guard rails” keeping it from misbehaving.
And the results confirmed our hypothesis. We did not see all broad match spending stop, and actually saw it grow over time. Now let’s look at the data, and see just how much spend from the broad match campaigns was negated by our scripting.
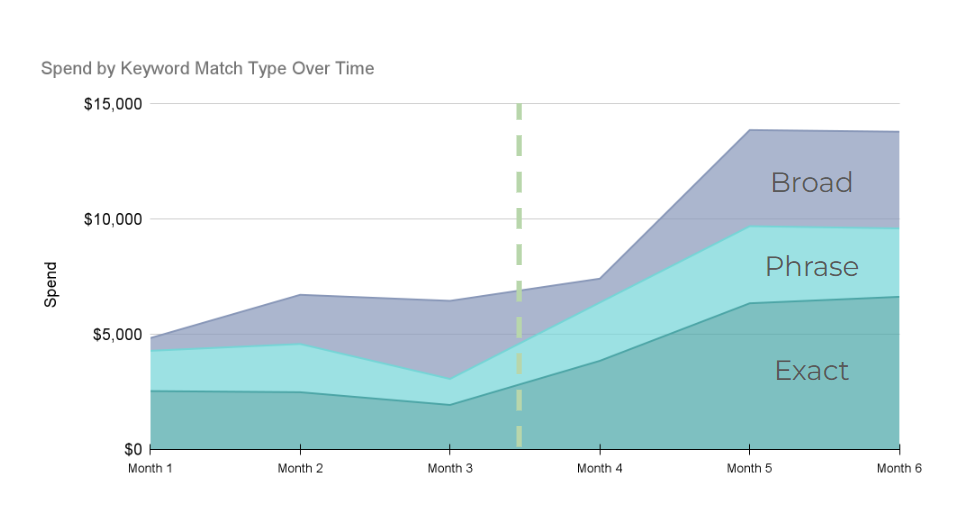
Initially, our campaigns faced significant challenges, with 23% of our total broad match spend coming from overlapping terms that could cannibalize more effective campaigns. To put this into perspective, our script was adding as many as hundreds of negative exact match keywords a day, every day. But it’s clear that the frequent addition of negatives allowed us to achieve remarkable improvements over a 10-week period.
As we navigated through this transformation, we observed a significant reduction in cannibalized spend—from 23% of all spend in the first week to just 1% by week ten—while simultaneously growing our overall campaign.
Over the course of 10 weeks, we saw the relative percentage of total spend going to these “best practice search terms” drop off steeply, stabilizing to lower levels by week 5! At the same time, we also saw consistent growth in relative and actual spend from our broad match cohort, indicating that we did more than just restrict and box in our broad match spending. Total spend across all keyword types grew by approximately 169.25% over 10 weeks, with an average weekly growth of about 18.81%.
At the same time, we saw a total drop of 22% in cannibalized spend over the 10-week period, declining from 23% in week 1 to just 1% by week 10. This significant reduction highlights the effectiveness of our automation implemented using the format outlined here (link to part of previous blog post)
And this is what our test delivered, from a keyword level spend perspective over the course of 6 months, with the before period in months 1 through 3 using Google’s recommended best practice, and months 4 through 6, using ours.
But the benefits of our new structure extend far beyond adding large numbers of negative keywords frequently through automation. We broadened our spend across keyword types, particularly increasing spend on phrase and exact keywords vs the time period when broad match was increasing at the behest of its counterparts.
The primary metrics we will be looking at here will be the ability of our campaign to generate traffic, and to improve our ROAS (Return on Ad Spend). What we want to see is growth in ROAS, and more efficient CPC’s both in aggregate and at the keyword level.
There is a negative correlation between performance and spend while scaling up Google Ads campaigns. Thus it is expected that we will see a slight reduction in metrics like ROAS as we increase spend. In order to measure the impact of our new strategy on our ability to scale, we will repurpose a metric more commonly used to predict whether a SaaS company has a profitable model for generating revenue; the Growth Efficiency Index.
Growth Efficiency Index
The Growth Efficiency Index (GEI) is a straightforward metric for understanding how well your campaign is scaling. When you push budget higher, it’s natural for ROAS to dip; GEI steps in to measure whether that spend increase is pulling in enough revenue to make the trade-off worth it. By calculating incremental revenue efficiency—revenue growth as a proportion of spend growth—and adjusting for the ROAS decline, GEI provides a clear picture of whether your campaign is scaling efficiently.

A positive GEI score means you’re pulling in strong incremental revenue despite the ROAS dip, indicating efficient scaling. If it’s negative, it’s a signal to revisit strategy before spending more. GEI is designed to give you a realistic, data-driven way to evaluate growth so you can maximize performance while scaling up. For our campaigns, a benchmark of 0 would be the minimum we would like to see.
With this in mind, our test yielded a GEI of .4, and we saw even stronger growth at the keyword level for phrase match keywords, which generated a GEI of 2.51 in the experiment.
Exact match keywords yielded a GEI of 0, indicating that there wasn’t much more room to grow, but this is also factoring in the fact that we saw a massive growth in exact match spend once we stopped broad match cannibalization, with 185% increase in exact keyword spend vs prior period. Thus we can conclude that we let exact match do exactly what it needed to do, to its fullest potential.
Broad match yielded a GEI of .16, which while positive was relatively low, aligning with the nature of the limited niche that broad match keywords are designed to fill, and their specific purpose of doing what other keywords cannot.
We took broad match, which when added to existing best practice campaigns had hardly any spend or conversion value, and doubled our broad match ST spend without major losses to ROAS.
Conclusion
In the end, broad match is just one of many new tools that are at our disposal as digital marketing experts. They can have numerous unique use cases, and this is a snapshot of how we were able to successfully test this new tool in a controlled environment. Over time, tools are introduced, grow in usage until they are widespread best practices, and eventually even become obsolete and get phased out.
In July of 2024, Google Ads changed broad match to be the default match type for search campaigns. Google states that this is part of efforts to leverage machine learning and automation to improve ad performance and simplify campaign management for advertisers. And that’s partly true, but the goals for Google, and the goals of managers of ad campaigns leveraging broad match keywords and search terms are not identical. Based on testing, our best practice is to launch new tests in a controlled environment where we can clearly and consistently measure the results. You can use our new structure in your own campaigns, and once you have the data, you can utilize the GEI in order to track performance of tests vs prior period, incorporating scale into that calculation.
Want to see how broad match can strengthen your campaigns? We’d be happy to dig in and discuss strategies. Reach out to us using our contact form or connect with us on LinkedIn.